Disclosure: Privacy Australia is community-supported. We may earn a commission when you buy a VPN through one of our links. Learn more.
Best Machine Learning Courses & Certifications in Australia
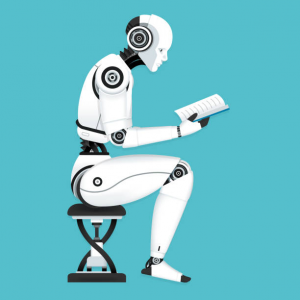
According to MIT Sloan professor Thomas W. Malone (the founder of MIT’s Center for Collective Intelligence, in the last five years or so, machine learning has become essential, and perhaps the most keyway, for most AI developments.
This means you’re often talking about AI when you talk about machine learning… and having skills in either will make you very valued as advances in these technologies take place.
These technologies are impacting every single sector, in the same way, that the internet or IT does. Regardless of whether you work in health or banking or defence, every field has been affected by IT, and in times to come, the same will be true for machine learning and artificial intelligence.
In this guide, we explore the best machine learning courses & certifications.
Table of Contents:
- Udemy Machine Learning A-Z
- Stanford Machine Learning Certificate
- Caltech Bootcamp for AI & ML
- Purdue University AI Machine Learning Certificate
- Kellogg Developing an AI Strategy Certificate
- Google Cloud ML Certificate
- Why Does Machine Learning Matter
- What is Machine Learning
- How Businesses Use Machine Learning Today
#1 Udemy Machine Learning A-Z
Udemy is one of the most popular and largest online course platforms available. You can find courses on just about any subject or topic including many different options for machine learning.
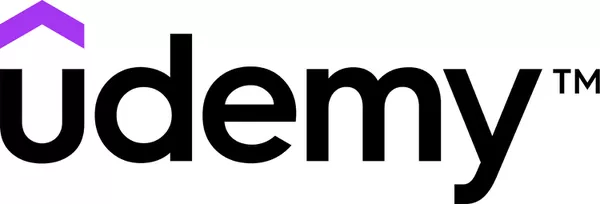
The course – Machine Learning A-Z has amazing instructors with strong reputations including Kirell Eremenko who is a data science consultant with over 1.6 million student enrollments. Hadelin de Ponteves is also an instructor who teaches in various machine learning fields, including computer vision and artificial intelligence.
There are 10 different sections of the course including data preprocessing, regression, classification, clustering, association rule learning, reinforcement learning, natural language processing, deep learning, dimensionality reduction, and model selection & boosting.
54% of the students that have completed the course gave it a 5-star review making it one of the top-rated machine learning courses.
Key Features:
- ☑️ Main instructors have solid reputations with decades of teaching experience in teaching machine learning and other similar topics.
- ☑️ Content is thoroughly structured to make sure you learn all important topics including data preprocessing, classification, and regression.
- ☑️ Great course for beginners since it starts with introduction modules to help you learn vocabulary and other important terms.
- ☑️ With over 700k students enrolled in this course, it’s one of the most in-depth options for those who are interested in machine learning.
- ☑️ Course content is broken into sections to make it easy to read through. You can also complete modules in your own time.
- ☑️ Kirill Eremenko’s teaching style is hands-on and very informative.
Length: 44 hours where you can work at your own pace
You can Register Here
#2 Machine Learning by Stanford University Certificate (Coursera)
The Stanford University Machine Learning certification is one of the most sought after largely because it is taught by Andrew Ng himself, once the head of both Google Brain and Baidu AI. For any ml certification to achieve a rating of 4.9/5 is no simple accomplishment – together with its sponsorship by Stanford University, this increases the program’s level of credibility.
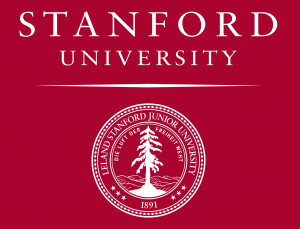
The program itself is based on a bunch of case studies and practical use cases, covering topics of Supervised, Unsupervised learning as well as bleeding-edge processes being used in machine learning today, in order to give you a sound foundation in how to use algorithms in order to develop intelligent robots, health analytics, database management, text and speech recognition, and other topics.
Key features:
- ☑️ A top recommended program to get to grips with machine learning methods used for business and daily living.
- ☑️ Covers a broad overview of machine learning, statistical trend recognition, mining databases, with all of the fundamental subjects.
- ☑️ Get up-to-date with the best practices in this field and most innovative breakthroughs being used by companies today for AI and machine learning
- ☑️ Learn how algorithms work with the creation of text recognition, test diagnostics, intelligent machines, audio, computer sight, and other topics.
- ☑️ Explore numerous lectures, video guides, hands-on exercises, and more in order to test and develop your understanding of key ml concepts.
- ☑️ Offers a professional certificate recognised by lots of employers by the program’s end.
Length: 2wks
You can Register Here
#3 Caltech Bootcamp for Artificial Intelligence & Machine Learning
Caltech CTME is aimed at professionals who already have data analyst skills and want to branch out into artificial intelligence and machine learning. This soup-to-nuts Bootcamp is a combination of IBM (who are deeply entrenched in the fields of AI) and Caltech (who have a strong academic reputation).
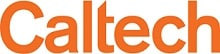
Caltech developed the course in tandem with IBM, giving students an online bootcamp where they can be exposed to lessons on neural networking, speech and text recognition, how computers visualise, and a bunch more. Classes use a mixture of lectures, quizzes, and use cases based on case studies of or how these industries are being used in business today. Case studies include more than two dozen practical exercises from firms like Hoover, Twitter, and more, which are also used to evaluate your progress.
Key USPs:
- ☑️ A comprehensive curriculum that teaches you all about machine learning and artificial intelligence in order to move your career into that pathway.
- ☑️ Make over 20 CEUs in the Caltech CTME Circle Members.
- ☑️ Discover all of the leading-edge best practices and technologies key to becoming an AI & ML pro – from a renowned Caltech academy.
- ☑️ Test out your level of competency and validate through earning a certificate from both IBM and another from Caltech.
Duration: Varies
You can Register Here
#4 AI Machine Learning Certificate (Purdue University; Post-Grad)
This particular program is only open to successful undergraduates aimed at giving your hands-on awareness of artificial intelligence and ML algorithms, in order to develop their optimal machine results along with the Simplilearn Bootcamp academic model.

To join this course, you already need to be an employed professional in this space with some basic programming abilities. The course will help you to develop them to work with NLP, statistics, machine learning, and more. Classes are taught mostly through interactive teaching sessions, some of these are live by industry experts, as well as building industry portfolios and more.
Once again, IBM has collaborated with Purdue University, which gives you access to a bunch of different industry leaders as you progress through the course.
Key USPs:
- ☑️ This comprehensive program gets you ready to handle tasks related to artificial intelligence technologies, such as deep learning (very important for self-driving cars), speech recognition, NLP and much more.
- ☑️ Develop Python programming using its many script libraries, including how to write scripts using the Jupyter lab context
- ☑️ Get educated on fundamental concepts of deep learning used in order to create artificial neural networks and investigate data abstraction including TensorFlow/Keras
- ☑️ Further expand your understanding of specific subcategories that you’ve taken special interest in, such as reinforced learning, GitHub learning, NLP and more.
- ☑️ Earn a professional certificate without leaving your house, according two your own pace and schedule of learning.
Duration: Takes a year if done for between 5 and 10 hours per week.
You can Sign up Here
#5 Developing your Own Artificial Intelligence Strategy (Kellogg Management School)
This particular program is business-oriented, as it’s acutely aware of the exponential adoption of Artificial Intelligence across industries in order to streamline and simplify administrative tasks.

Cloud computing and big data have already required an enormous amount of data analytics. AI is furthermore transforming how organisations go about their operations and serve customers. So this course by Kellogg School of Management is made for leaders and executives looking to adopt AI in their business.
The course is taught using a bunch of interactive and live sessions, in accordance with hands-on exercises that use more than 50 real-world examples. Change leaders will go through live datasets in order to get a practical understanding of AI integrations in order to modify the experience of the consumers and for improving business operations.
Key USPs:
- ☑️ Understand how your organisation can be scaled up using streamlining AI integrations.
- ☑️ Grow awareness of integrating AI through the lens of practical use cases used in leading companies across industries.
- ☑️ Have flexible access to the program through your mobile including to lectures, when on the move.
- ☑️ Learn about key patterns and trends, as well as services that are currently spearheading AI-powered business operations.
- ☑️ Increase the fitness of your customer support structures & customer experience through AI programs.
- ☑️ Earn a Digital Certificate once you pass the exam with an 80% or higher score.
- ☑️ Be a change leader in your firm to integrate AI-driven business plans.
Duration: 60 Days
You can Register Here
#6 Google Cloud Platform: Machine Learning Via TensorFlow (Beginner-Friendly, on Coursera)
A mind-boggling 2.5 quintillion bytes of information is being generated on the Internet on a day-to-day basis. There’s no doubt that an unfathomable amount of valuable insights and uses can be extracted from this ocean.
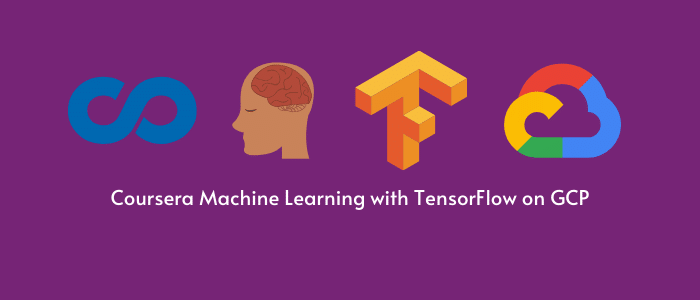
Made up of five programs, this curriculum aims to give students a firm overview of the value of machine learning today, through lectures based on developing ML models. The course begins with entry-level lessons that describe the capabilities of machine learning and why it is growing in popularity, then the course goes into Tensorflow, which is a machine learning model that is open-sourced.
This collection of lectures is designed to help you with developing, training, executing ML frameworks, solving numerical tasks, and more. There are also lots of practical opportunities for learning how ML accuracy can be increased through the various offerings on the Google Cloud Platform.
Key USPs:
- ☑️ Overviews critical machine learning concepts and the most valuable use cases, for problem solving.
- ☑️ Focuses on developing distributed machine learning frameworks that build up in in TensorFlow, and how to streamline developing training for these models on a bigger scale.
- ☑️ Investigate the optimal configurations by playing with parameters in order to generate the most accurate, applicable models as well as understanding the concept.
- ☑️ Improve your capabilities with practicals that take place on the Google loud platform.
Duration: Between 1-2 months at 8-10 hours per week
You can Register Here
Why Does Machine Learning Matter?
Machine learning makes predictive text, chat box, speech recognition, language translation tools, recommendation feeds, and more possible. Machine learning is also behind self-driving vehicles and diagnostic machines needed for detecting health conditions based on visuals and scans.
In fact, when an organisation uses an artificial intelligence program, they’re usually also using machine learning – to the degree that the two terms are frequently interchangeable, and sometimes mysterious. Machine learning is a subset of AI focused on giving machines the capability to self learn without needing to be programmed.
This is used everywhere from manufacturers and retailers and banking to fishmongers. Even traditional firms are adopting machine learning in order to discover new opportunities, optimise efficiency, or find hidden value. One MIT computer scientist who is also an MIT professor, Aleksander Madry (and MIT Centre for Deployable Machine Learning director), believes machine learning will alter every industry, which means that executives and decision-makers in the sectors need to understand machine learning.
Although everyone needs to know the technical ins-and-outs, they need to understand the capabilities of this technology. Few can afford to not be aware of what lies around the corner.
This extends to ethical, social, and national impacts of machine learning. It’s crucial to begin developing an understanding of how these tools work, and how to use them.
So, What is machine learning?
Once again, ML is a subset of AI, which itself can be generally defined as the capability of a machine to mirror intelligent human behaviour. Artificial intelligence programs aim to carry out complicated tasks in a similar way to how humans tackle problems.
The objective of AI it’s a develop computer systems that demonstrate “intelligent behaviours” in the way of humans. An example of this is computer vision, which means the ability of a machine to recognise a scene visually, or to carry out a specific task on a physical plane, or to correctly understand naturally written text.
So machine learning is one way to put AI to use. It was coined in the 1950s by one AI innovator Arthur Samuel as a field of study focused on giving computers the capability of learning without explicitly being programmed.
Today, this definition seems to hold up, with one professor Mikey Shulman who teaches at MIT Sloan is also Kensho’s head of machine learning (this company develops artificial intelligence for financial and intelligence organisations in the U.S.). Mikey thinks of the old way we went about programming machines as like a baker who needs very exact amounts of ingredients and a specific amount of time in order to bake anything. Every instruction programmed into the machine has to be explicit and specific.
But trying to apply this approach to more complicated tasks that batch a bunch of other tasks with a lot of variance, such as trying to recognise pictures of people as compared to pictures of objects, would be either impossible or extremely time-consuming if written in the traditional programming way. By comparison, machine learning teaches computers to program themselves by being exposed to these tasks and being validated along the way.
How Businesses Use Machine Learning Today
ML is already a fundamental component to many businesses today, such as YouTube’s suggestion algorithms or the Google search engine itself. A number of other businesses are making contact with machine learning, but are not yet making it the central business model.
While several others are considering ways of using machine learning for the greater good. This in particular is the most difficult challenge for this technology, there is an immense chasm of knowledge of how machines can solve some of the biggest human problems, or even how they can integrate socially as they become more powerful.
In a notable study conducted in 2018 by researchers at the MIT Initiative on the Digital Economy, a comprehensive evaluation of machine learning’s applicability was undertaken. The researchers devised 21 fundamental questions to assess the suitability of tasks for machine learning processes. Remarkably, they concluded that no industry or job would be exempt from the influence of machine learning. However, they also found that no single job would be completely replaced by machine learning.
According to these researchers, machine learning will prove most effective in handling discrete tasks. Some of these tasks may only be achievable through the application of machine learning, while others are presently performed by human workers. This suggests that machine learning will augment and complement human capabilities rather than fully supplant them.
The findings of this study highlight the potential of machine learning to revolutionize various industries and job roles. By identifying tasks that can be enhanced or automated through machine learning, organizations can harness the power of this technology to improve efficiency, accuracy, and productivity while also leveraging the unique strengths and expertise of human workers.
Today, businesses are already incorporating machine learning in the following ways:
#️⃣ Algorithmic recommendations. In short, suggestion feeds, such as those used by social media or Netflix and YouTube. Today, the bulk of information that shows up on your devices is powered by machine learning. These algorithms are trying to figure out our preferences. Programmers are teaching them to learn what users enjoy, such as on Twitter. What do you want our devices to show us, what posts did we like, what content did we share?
⚠️ Computer vision. Used for detecting objects and people. Machine learning can analyse images in order to scan for different datasets, such as telling apart different people – this uses facial recognition, some of which is controversial. Business use cases for this capability varies. Some of it is downright surprising, for instance some hedge funds use machine learning in order to analyse how many cars were in parking lots, in order to figure out whether companies were actually making good bets and performing well.
👮 Detecting fraud. ML is (arguably) very good at analysing patterns and trends, as well as spotting deviations from these. This is used in order to spot potentially fraudulent transactions, such as for credit cards, or spamming, or unauthorised logins.
💬 Chatbots. Automatic helplines and chatbots have been growing in the last couple of years. Customers are outsourcing their support offerings to these machine scripts, making them the first point of contact with troubleshooters – these programs use algorithms in order to interpret natural languages and to process them, and are able to learn from archival conversations. And hoping to give the right right response.
🚗 Autonomous vehicles. Also known as self-driving cars. These depend on machine learning and especially rely on deep learning.
😷 Making medical diagnostics and imaging. Finally, ML useful programs that analyse medical images or other datasets in order to find specific markers indicating ill health, such as tools that can predict the probability of cancer-based on a mammogram.
You Might Also Like: